Spectacular Tips About Why Choose The Arima Model Types Of Line Graphs In Math
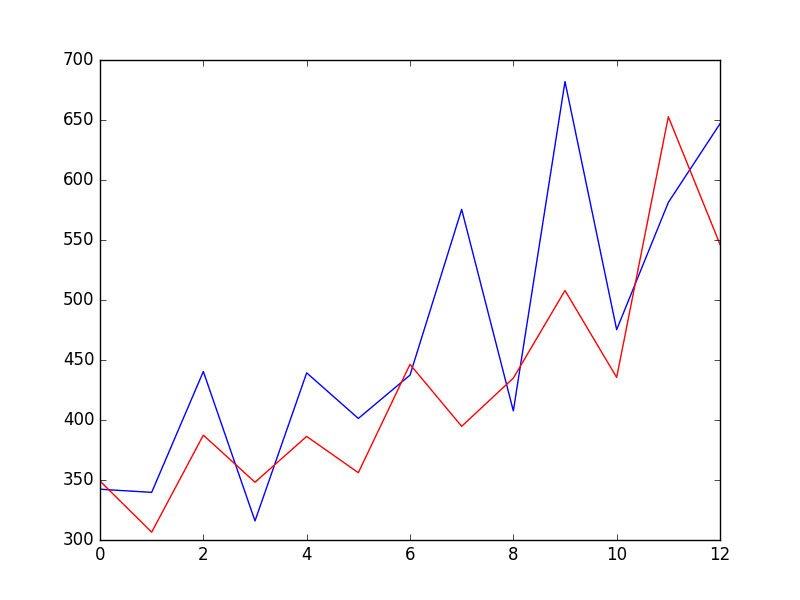
I have tried but still unable to identify which arima model is appropriate for my data set?.
Why choose the arima model. Sometimes an ar model provides an adequate representation of the data generating mechanism. Examples and applications of arima models. Autoregressive integrated moving average, or arima, is one of the most widely used forecasting methods for univariate time series data forecasting.
Arima is one of the fundamental time series. Other times an arima model is more appropriate. Arima models provide a robust framework for analyzing and forecasting time series data.
It is a class of statistical algorithms that captures the standard temporal dependencies that is unique to a time series data. Arima models are a powerful tool for analyzing time series data to understand past processes as well as for forecasting future values of a time series. Identification and specification of appropriate factors in an arima model can be an important step in modeling as it can allow a reduction in the overall number of.
Arima models are not generally preferred over any other time series analysis method. If you want to choose the model yourself, use the arima() function in r. Choosing your own model.
There is another function arima() in r which also fits an arima model. In this tutorial, you’ll learn how to build arima models for time series prediction, with an example in python. I am doing my project on forecasting and i have to use the arima for it.
By incorporating autoregressive, integration, and moving average. What is arima modeling in short? Model and predict the dependence.
Arima models are, in theory, the most general class of models for forecasting a time series which can be made to be “stationary” by. An autoregressive integrated moving average (arima) model is a statistical tool utilized for analyzing time series data, aimed at gaining deeper insights into the.