Favorite Info About What Does An R2 Value Of 0.9 Mean Add Static Line To Excel Graph
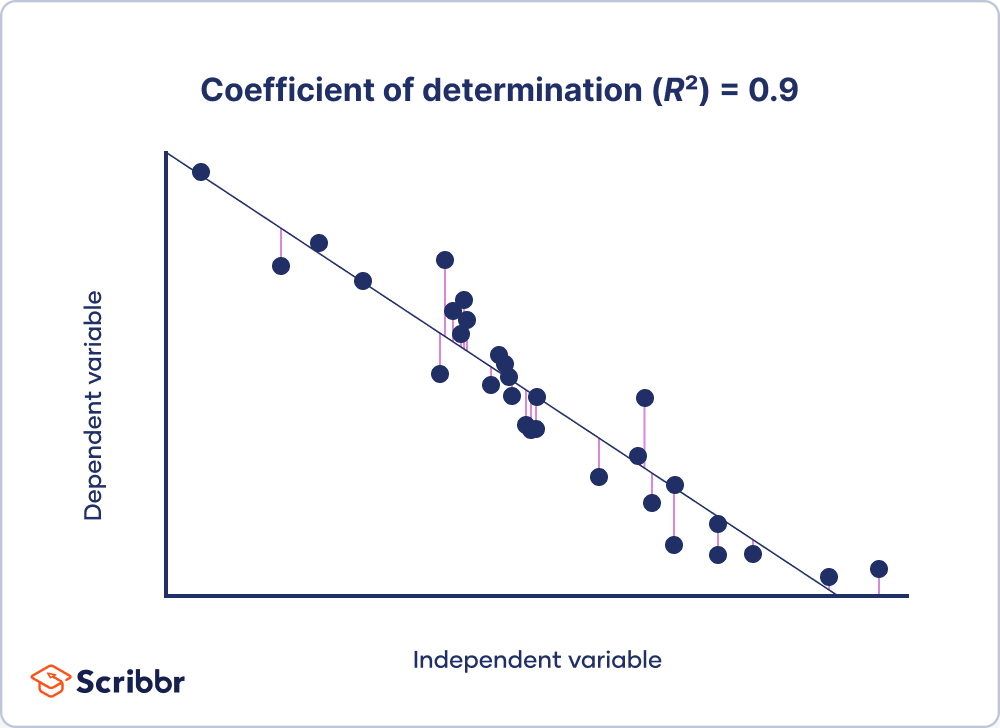
0% indicates that the model explains none of the variability of the response data around its mean.
What does an r2 value of 0.9 mean. 100% indicates that the model explains. The immediate question you may be asking: It seems pretty remarkable that simply.
Consider what $r^2$ means: A value of 1 indicates that the response variable can be.
An r2 value of 0.9 means that the independent variable accounts for 90% of the variability in the dependent variable,. R 2 varies between zero, meaning there is no effect, and 1.0 which would signify total correlation between the two with no error. Proportion of variability explained, compared to a baseline model that always guesses the average value of the pooled response.
The coefficient of determination ( r ²) measures how well a statistical model predicts an outcome. The sum of squares of the residual errors is far greater. What does a 0.9 r2 value mean?
R squared measures how much of the dependent variable variation. What we usually observe are values ranging from 0.2 to 0.9. R squared (also known as r2) is a metric for assessing the performance of regression machine learning models.
What does an r squared value mean? A value of 1 indicates that the response variable can be. I'd expect the r² score to be very low, because it clearly isn't a good prediction, but.
The outcome is represented by the model’s dependent variable. Unlike other metrics, such as mae or rmse, it is not a measure of how accurate the predictions are, but instead a measure of fit. How do i know which is better?
Many formal definitions say that r 2 tells us what percent of the variability in the y variable is accounted for by the regression on the x variable. A negative correlation coefficient means that the relationship between the variables is negative (also known as “anticorrelated”, which is not the same as uncorrelated!). What does an r2 value of 0.9 mean?
It is commonly held that higher r 2 is better,. A value of 0 indicates that the response variable cannot be explained by the predictor variables at all.